In Person Only at the Boston Park Plaza
9 – Machine Learning Methods for Precision Manufacturing Applications
Dr. Satish Bukkaptanam, Yuhao Zhong & Tapan Ganatma Nakkina (Texas A & M)
Monday, November 13, 2023
8:30 AM – 12:30 PM (EST USA)
Overview: This tutorial aims to provide participants with a comprehensive understanding of the taxonomy of machine learning (ML) methods relevant to the precision manufacturing field, focusing on their application workflows and problem-solving capabilities.
Target Audience: Professionals and practitioners in the field of precision manufacturing with an interest in ML methods and their application workflows.
Tutorial Objectives
- Introduce participants to the taxonomy of ML methods and concepts relevant to precision manufacturing applications. This includes an overview of 4 major ML categories: supervised learning, unsupervised learning, semi-supervised learning and reinforcement learning.
- Provide an overview of the different workflows involved in applying ML methods to solve precision manufacturing problems. Generally, the workflow includes data preprocessing, model generation, model selection and model evaluation.
- Showcase case studies highlighting successful machine learning applications in precision manufacturing, demonstrating the breadth of problem-solving capabilities.
- Foster interactive discussions and Q&A sessions to address participants’ specific interests, concerns, and practical challenges in applying machine learning in precision manufacturing.
Tutorial Agenda
- Introduction to Machine Learning in Precision Manufacturing
-Definition and scope of machine learning in the precision manufacturing domain
-Key benefits and challenges of applying machine learning in precision manufacturing
-Taxonomy of Machine Learning Methods and Concepts - ML Application Workflows
-Workflow templates based on different manufacturing topics, including but not limited to predic1ve maintenance, quality assurance, process op1miza1on, fault detec1on and root cause analysis.
-Workflow templates based on different input data types, i.e., tabular, images, textual, and graphs. - Case Studies
-Research outcomes from our group with a focus on defect detection/localization.
-Toy examples in other selected topics - Q&A
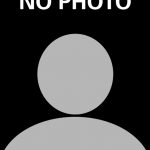
Dr. Satish Bukkaptanam Dr. Satish T. S. Bukkapatnam’s research in smart manufacturing addresses the harnessing of high-resolution nonlinear dynamic information, especially from wireless micro-electromechanical systems sensors, to improve the monitoring and prognostics, mainly of ultraprecision and nanomanufacturing processes and machines, and wearable sensors for cardiorespiratory process prognostics. His research has led to over 160 peer-reviewed publications (101 published/accepted in journals and 68 in conference proceedings); five pending patents; $6.5 million in grants as PI/Co-PI from the National Science Foundation, the U.S. Department of Defense and the private sector; and 17 best-paper/poster recognitions.
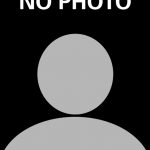
Yuhao Zhong
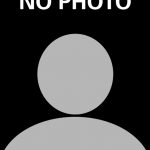
Tapan Ganatma Nakkina